Beating the bottlenecks: the power of analytics in manufacturing
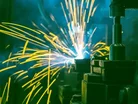
Ruban Phukan, Co-founder and Chief Product and Analytics Officer at Progress DataRPM, discusses the age-old battle with bottlenecks and the role of predictive analytics in manufacturing.
The issues surrounding bottlenecks in manufacturing are well documented. From production delays, overstock, increased pressure from customers and beyond, the problems caused by a sudden, unforeseen limitation in capacity can be devastating for any business in the industry. With this in mind, Manufacturing Global speaks to former Yahoo data scientist and leading light at Progress DataRPM, Ruban Phukan, about the use of predictive maintenance models and how they can help establish a fail-proof environment.
Getting to the heart of the problem
As a former member of the Yahoo data analysis team, Phukan is well versed in the complications surrounding the use of big data. “I was part of the first data science team that was created at Yahoo many years back,” he says. “The big learning there was that data science is very hard to scale, manually, for large organisations. What we realised is that the only way to really solve the data science problem, in a manner that can add value to a business, is to automate the processes that go behind that data analysis.”
Moving on from Yahoo, Phukan started his own vertical search engine business, using machine learning to understand user behaviour, specifically dealing in predictive analytics. After selling it on he went into business with his current co-founders, which is where he picks up the story of ProgressDataRPM and how it is making strides towards, among many other things, tackling bottlenecks.
“When we first got together we realised there was a huge amount of digital data that was being generated, but the problem remained the same: it can’t be solved manually. There are just not enough data scientists. Even with the huge amount of data out there, the kind of value businesses were getting out of it doesn’t justify the ROI,” he says.
“So, we started saying ‘can we create a platform that makes data science processes seamless and automated?’ We wanted businesses to be able to leverage best practice, apply it to their problems and solve it at scale.”
Advances in machine learning
With the advance in the technological capability of machine learning, Phukan and his co-founders were able to address the sheer volume of data and information available to their clients in a way that wasn’t possible before. Crucially, for manufacturing businesses, this meant they could also initiate a positive impact on things such as the production line.
“For example, with things like sensors being enabled, it helps us understand at a very detailed level, the normal functioning of a machine under different operating and environmental conditions. We can then assess how this affects a machine’s health and consequently what this does to the production line, in terms of efficiency,” he tells us.
By deploying an automated plant farm that doesn’t require someone to be a data science expert, ProgressDataRPM essentially helps large asset-based industries to solve two problems: how to minimise unplanned downtime and how to maximise the quality and efficiency of the output of their assets. This is all done via its cognitive anomaly prediction software. The moment it’s connected to sensor-based data it automatically baselines the normal operating conditions of the machine. It then looks for things that deviate from these conditions and creates an alert before predicting the potential issues that might occur.
It’s this ability to predict the unknown that Phukan believes represents a major departure from previous traditional processes. “We need to be able to examine why things have happened in the past but, more importantly, we need to also predict things that haven’t happened,” he explains.
Impacting the assembly line
One of the key areas that manufacturing businesses need to examine are the processes that impact what happens on the production line, specifically the timing of quality control. “We’ve worked with a number of manufacturers in the automotive and healthcare industries, for example, and one of the biggest challenges they face is that the quality check happens at the end of the manufacturing process. So, once a whole batch of a product is made, the tests will be run to determine whether it’s OK. This can lead to scrap rates as high as 75%,” says Phukan.
As most test suite rules can only be written based on previous experience, when a new problem arises that hasn’t been accounted for, this can lead to serious issues like product recalls. Using predictive analytics platforms will therefore, according to Phukan, become the norm. Indeed, this is something he’s already identified increasingly in manufacturing.
“When manufacturers speak to us, they ask if they can use machine learning to determine, along the way, if a batch is defective. They know we can detect things much earlier, so they can stop the process to minimise cost and loss.”
Does he have any specific examples of this in action? “We work with a large telecom provider in France and they had a real challenge with their set top box, which is not something you would assume requires heavy predictive maintenance. The problem they had was that every time one failed they had to go and identify the issue and replace it. Obviously, this is costly in terms of time and money. There’s also a huge risk of losing customers,” he says.
“We proactively identified that one third of their boxes had a problem which meant customer service could call and tell the people to update their box or send a replacement before a failure occurs.”
The future of bottleneck analysis
Manufacturers are clearly becoming more attuned to the benefits of machine first cognitive technology, which allows them to identify anomalies and potentially bring in a subject matter expert to help determine the ramifications of the machine’s findings.
Indeed, Phukan discusses how AR, IoT, warranties, hybrid cloud computing and blockchain will all increasingly play their role too, however, it’s detecting the unknown that he feels will be key. “The key is not what is already known; domain experts can do a great job handling that, but the big challenge is the unknown and what’s going to hit businesses next. That’s what we need to prevent and that’s the crux of what will change the manufacturing game,” Phukan concludes.