GE Digital: smarter manufacturing with AI & analytics
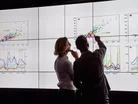
Cobus van Heerden, Senior Product Manager, Analytics & Machine Learning, GE Digital, discusses the current AI and analytics trends in manufacturing.
What are the current trends within manufacturing when it comes to AI and analytics?
Process engineers have exceptional domain expertise to put together process models – or Process Digital Twins – and be able to interpret the models. This is the foundation for improving competitive advantage and success with analytics.
What are the benefits of AI in manufacturing relating to analytics?
With Machine Learning and analytics, industrial organizations can capitalize on the IoT opportunity, optimize operations, and generate greater profitability. Additionally, engaging in the latest analytics technologies also helps to attract and retain the best talent.
What are the challenges of AI?
All process engineers can and need to develop capabilities in analytics and machine learning to remain competitive – both at an individual professional level as well as to help their industrial organization – in our world of digital transformation.
Over time, engineers can go from small projects to pilots to multi-plant optimization with deep application of analytics. Engineers’ deep domain expertise provides a foundation for modelling processes and developing the analytics that are game changers in very specific applications. The combination of applied analytics technology with those Process Digital Twin models uncovers hidden opportunities for improvement over and over again.
What is the best strategy for driving the best business value from AI and analytics when it comes to analytics?
Fortunately, the journey to success with machine learning and analytics doesn’t mean that process engineers suddenly need to become data scientists. Proven processes and software technologies make analytics achievable for every industrial organization. To support the journey to machine learning and analytics, companies like GE Digital provide analytics technology training in the form of a self-serve product university, detailed demo videos, and application advice.
Additionally, while today’s software features enhanced ease of use and no-code implementation extensible with Python, process engineers can still lean on product experts in combination with their own domain expertise to mine data and leverage analytics to improve operations.
To drive analytics and improve processes, organizations can align domain expertise and strategically drive analytics in five areas:
-
Analysis - automatic root cause identification accelerates continuous improvement
-
Monitoring – early warnings reduce downtime and waste
-
Prediction – proactive actions improve quality, stability, and reliability
-
Simulation – what-if simulations accelerate accurate decisions at a lower cost
-
Optimization – optimal process setpoints improve throughput at acceptable quality by up to 10%
Looking to the future how would you like to see this technology developed within the industry?
Software solutions using advanced AI and machine learning to enable process engineers need to continue to be innovative and outcome oriented. These solutions need to focus on providing the ability to combine data across industrial data sources and rapidly identify problems, discover root causes, and automate actions to continuously improve quality, utilization, productivity, and delivery of production operations.
SEE ALSO:
- Top four enablers for digital and analytics transformation
- Manufacturing Analytics Market to see huge growth by 2030
- Tableau: innovating the way organisations harness data
- Read the latest issue of Manufacturing Global here
For more information on manufacturing topics - please take a look at the latest edition of Manufacturing Global