Tackling the manufacturing challenge with data and AI
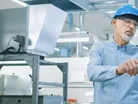
“In manufacturing, you're under pressure to continuously improve quality while reducing costs and increasing productivity,” says analytics software and solutions leader SAS. This remains as true as ever in the digital age, and technological advances have conspired to tackle the challenge more effectively than ever before.
Even a cursory glance makes it plain that, of all the industries to be shaken up by digital transformation, few have been transformed more visibly and dramatically in recent years than manufacturing. This journey, from Henry Ford’s workforce-intensive production lines to the increasingly people-free environments of modern factories, has been heavily accelerated by the advent of Big Data. Harvesting information from manufacturing operations, equipment and machinery, sales patterns and demand fluctuations enables leaders to strategise for greater efficiency, output and safety. The Industry 4.0 vision of smart factories that run with minimal human input whilst yielding higher productivity, profitability, and reliability is enormously attractive, but realising this goal brings equally sizeable challenges that must be overcome.
The data wave
Back in 2018, leading consultancy and outsourcing services provider Capgemini identified manufacturing as having among the most considerable potential for operational improvement through Big Data, going as far as to say that its adoption is vital for continued business success. “With Big Data analytics no longer being a ‘nice to have’ option, companies must identify the right opportunities to improve plant efficiency and generate insights,” Capgemini said in a November 2018 blog post. “Big data analytics would then provide the competitive edge companies need to succeed in an increasingly complex environment.”
With vast volumes of data - a mass that increases exponentially for operations making use of Internet of Things (IoT) powered technology and the gigabit-per-second transfer speeds of 5G - comes immense complexity. Not only must organisations zero-in on the value of a broad data set, but they must turn that nascent value into actionable insights before they’re superseded by newer and more relevant data. Often, raw data must be married with the telemetry of other systems for its worth to be extracted, and this know-how on a human level is difficult to come by.
The case for AI
Around the globe, trained data scientists are in high demand and short supply and, even with a fleet of top data analysts, the true value of an organisation’s data is locked behind the lengthy time for complicated analyses to be undertaken, the likelihood of human error, and the need for deeply considered data implementation strategies. The need for immediacy and accuracy, particularly with the widespread data skills gap, can be answered by another emergent technology: artificial intelligence (AI).
Its algorithmic calculations and analyses tailorable to the needs of specific operations yield results that are both more accurate and rapidly available, enabling enterprises to turn that data into informed action that improves efficiency and productivity, scales operations to real-time demand and improves safety, uprooting the problems posed by a shortfall in expertise whilst building value. It can even catch problems before they arise through predictive maintenance, something that would require a considerable labour force and time allocation without the promise of accuracy that AI provides.
“Predictive maintenance is one area where IoT, Big Data and analytics are making a significant impact,” says Debbie Heaton-Bowen, Partner at Oliver Wight EAME. “Although it originated in the 1990s, the advent of advanced technology has meant that the capabilities of predictive maintenance have recently been ‘supercharged’, particularly in the manufacturing sector with smart factories becoming a reality. Unplanned downtime and poor maintenance can cost companies millions, but IoT-enabled sensors can detect when machinery needs a check-up, preventing the development of a more serious fault which would cause costly disruption. Not only does predictive maintenance identify errors missed by the human eye, but it also makes solely data-driven decisions to improve the lifespan of machinery, lower service costs and increase operational efficiency for healthier profits.”
Elsewhere, robotics are another trend in the industry that shows considerable promise, and AI is vital to their value proposition when deployed across manufacturing processes. “When integrated correctly, these robotic units can amplify people’s skills and strengths to increase workplace efficiency and improve the employee experience,” says Prasad Satyavolu, CDO of Manufacturing and Logistics at IT services leader Cognizant. “They are already being deployed across manufacturing organisations, from inserting shock absorbers or cutting meat on a traditional assembly line, to drones acting as the eyes of security officers patrolling a vast container yard. In doing so, the new breed of autonomous co-workers is freeing up humans to take on higher value work. Researchers at MIT found that human-robot teams working for BMW were approximately 85% more productive than humans or robots working alone.” AI can thus simultaneously bridge skills gaps and maximise the time available for skilled workers to focus on tasks where they can add more value.
The challenges of AI uptake
Despite the clear opportunities it presents, management consultancy McKinsey notes that AI uptake has been notably slow in the manufacturing industry. “While AI technologies have made tangible improvements to supply chains and administrative functions, they have so far had scant presence in production,” McKinsey says, noting that this slow adoption has been triggered in part by a heavy reliance on expertise and knowledgeable workforces, which is somewhat ironic given the capacity for AI to redirect this expertise to less repetitive processes. The reliance on skilled workforces in itself is cause to accelerate AI’s integration in the industry.
“Since variations in operators’ qualifications can affect not only performance but also profits, AI’s ability to preserve, improve, and standardise knowledge is all the more important,” McKinsey says. “Moreover, since it can make complex operational set-point decisions on its own, AI is able to reliably deliver predictable and consistent output in markets that have difficulty attracting and retaining operator talent.”
Along with this reliance on human capital, many factories rely on legacy machinery that predates even the internet, and those introduced in the past two decades require some measure of modification to make them compatible with the data-harvesting tech of today. Meanwhile, the questions of sourcing the most impactful data sets, how best to interpret them and implement the findings vary not only by company but by departments and teams as well.
Research firm NelsonHall notes that the adoption of MES (Manufacturing Execution Systems) increases this complexity further. MES has been enabling firms to digitise their operations and access data more readily for years, but the deep customisation of each facility’s own MES means compatibility and integration with other technologies, such as the analytics software that collates and analyses data from myriad sources, is a considerable challenge. “The difficulty that MES brings is that it is difficult and costly to upgrade, given the level of customisation,” says NelsonHall. “Also, MES governs the production of a plant, so they are critical systems and therefore require rigorous development and implementation, testing, and roll-out. In other words, they are comparable to mainframe applications: as long as they are running, no one really wants to touch them too deeply.” Circumventing this problem is thus often done through the addition of complementary systems, such as that provided by Dassault, Siemens, PTC and the like, adding yet more complexity where many operators are seeking to streamline and slim down their tech infrastructure to increase flexibility and agility.
Tackling the challenge hydra
Clearly, the challenges of successfully harnessing manufacturing data sets are huge: strategy, telemetry, integration, expertise, and legacy infrastructure must each be addressed to facilitate a seamless move to Industry 4.0. AI alone, sadly, cannot solve the headaches of successfully implementing the data-based technologies that will drive manufacturing into Industry 4.0. Industrial IoT, robotics, digital twins, and predictive maintenance have all rapidly gained traction as powerful modernisation tools for manufacturing, each made possible by Big Data and AI, but as with their forebears they each require considerable strategic consideration to be truly successful. The human touch, and operational alignment, are key.
“A successful data-driven transformation strategy needs to consider a comprehensive view of various factors including organisational culture, customers, employees, and technology. This cannot be achieved through isolated initiatives, and instead needs concentrated focus, medium to long-term planning, sponsorship, and investment directly from business leaders,” says Rohit Gupta, VP and Head of Manufacturing, Logistics, Energy and Utilities at Cognizant. “Manufacturers need to assess their current level of digital maturity, understand the business and technical challenges, and clearly envision a roadmap to thrive in this digital paradigm. This must be an iterative approach, working towards developing a foundation that can support scalable and sustainable growth and build data capabilities within the organisation with clear milestones.”
Outside help is a huge accelerator, and SAS, McKinsey, and Capgemini to name but a few have done much to bridge the technological and strategic gaps of enterprises and manufacturing powerhouses looking to make the leap into the smart future. After all, today’s tech ecosystems are predicated on a bigger focus on outsourcing and the knowledge that outside expertise is often superior in a tech space that gets more granular by the day. Manufacturing is deeply complex and one size can never fit all, but leveraging the expertise of leaders across the data and AI spaces is sure to overcome the challenges posed and unlock the enormous potential of Industry 4.0.
- Schneider Electric: Powering Manufacturing Via Cloud DataDigital Factory
- LG Electronics Enters Smart Factory Market with AI TechSmart Manufacturing
- Key Themes at Manufacturing & Mobility LIVE 2024Production & Operations
- Dassault Systèmes & Mistral AI Advance Generative EconomyAI & Automation