TIBCO on manufacturing inside the new anomaly economy
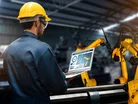
The way we use data to monitor and control manufacturing systems has changed. TIBCO’s director of digitalisation strategy Alessandro Chimera puts on his electronic overalls and recharges his digital grease gun to explain the shape of the anomaly economy guiding operational performance management and discusses everything from digital twins to AI, automation to human oversight.
Some parts of the manufacturing industry still exist as they did at the turn of the 1900s. We still need the grease, gears and grinding machines alongside the overalls and tea breaks. Those fundamentals will probably pervade far into the automated autonomous future because humans will always play a part in the wider foundational narrative that operates any manufacturing facility.
But, of course, we do know that manufacturing has changed. Throughout the first, second and now third industrial revolutions we have seen steam-powered acceleration, mechanisation and now IT-driven automation applied to manufacturing operations in ways that our predecessors would never have imagined possible.
As we enter the hyper-controlled manufacturing facility of tomorrow with digital twins and data now joining our physical machines and human workers, we must ask ourselves how we are to approach anomaly detection. It’s fairly easy to spot a dip or a spike on a graph that reports a significant measurement, but what do we do when there’s a slow degradation of quality or performance that happens over a month, or perhaps longer?
Blinded human oversight
Modern manufacturing facilities now operate at a mass-production scale that outstrips any single person’s (or even group’s) ability to be at every point on the factory floor at once. In these environments, we can not trust human observation to provide us with the complete picture that we need. In today’s modern facilities, there is rarely just one variable to track, it’s more likely to be hundreds or thousands of variables.
If a production anomaly ‘creeps’ into our operations slowly, it may go unnoticed until it’s too late and we can’t do anything about it. We need to talk about new ways of working.
A needle in a manufacturing data haystack
We know that industrial datasets are very large and extremely complex. Detecting and predicting anomalies is therefore harder than searching for a needle in a haystack as we often don't know what we’re looking for. But, detecting an anomaly means savings, which in turn translates into higher production yields, both immediately and further down the line.
Many manufacturers know that they have massive datasets, but all too often those data stores are siloed and scattered across multiple systems. This dispersion and divergence of data makes it hard to understand when an anomaly is related to multiple data systems, or related to different physical production phases. Indeed, sometimes it is necessary to retrieve data from products that are already on the market that have exhibited operational or functionality problems. Either way, the first step is to unify all your data sources.
Today we work in a world where analytics is becoming a fundamental part of modern factories; a trend underlined by the development of digital twins, where we need to apply accurate, rapid and intelligent analytics to gain the predictive insight that these virtual machines can provide.
Once data is available and can be accessed, advanced analytics is there to start analysing what to look for. Modern data science techniques are helping us to discover hidden anomalies that aren’t immediately understood due to the enormous number of parameters that can influence product quality.
Machine learning and AI scouts anomalies
This brings us to today. We are now at a point where machine learning (ML) and artificial intelligence (AI) are making their way into the production process as well.
Some manufacturers have initially used ML to get an understanding of their marketing initiatives, segment customers and trigger specific actions. But in more recent years, the more progressive and advanced manufacturers have started to understand the benefit of using ML and AI techniques to detect anomalies.
One example is the use of autoencoders to learn from data. An autoencoder is an artificial neural network intelligence technique used in ML. It is used to code and feature-learn from unlabeled datasets in what we commonly call unsupervised learning.
Our real-time efficient manufacturing future
By applying trained statistical models to real-time data, it is possible to predict when an anomaly will occur. This drives us to a new point of efficient operations. All of which leads us to the point where we can now shift from reacting to an anomaly, to predicting the anomaly with the benefit of achieving substantial operational cost reductions. We reduce costs as a direct result of reduced defect and scrap rates and prevention of unplanned equipment downtime.
Hemlock Semiconductor (HSC) is one leading manufacturer that utilises process control, predictive maintenance and anomaly detection solutions to streamline and control its semiconductor manufacturing.
By introducing near real-time alerting for individual manufacturing processes, employees can now automatically compare key parameters against pre-defined thresholds, statistical rules and optimal patterns uncovered through machine learning and AI methods. HSC receives automatically generated alerts as soon as a process falls outside of acceptable parameter bands, notifying manufacturing personnel that something requires their attention. Personnel can then readily access data to see precisely which variable may have caused the problem. Once these cause-and-effect relationships are identified, teams take action to prevent process defects from happening again.
All of this progress ultimately leads us to our tomorrow and the future of our planet. As the use of digital twins expands in line with the application of data analytics and ML, every manufacturer becomes a data-driven factory, focused on increasingly precise anomaly detection. At this point, manufacturers can work more acutely with optimised energy consumption for the good of us all.
Plant productivity will always be front of mind and as the future is undeniably data-driven, this is a path that we must logically take. As a result, we will be able to put products on the market that deliver not just better performance, but also work better and last longer. What impact that has on the cheap disposable goods market – where quality is tuned to ‘expire’ after a given period of time - is another question for another day.
The way we manufacture goods and related services can now be improved through anomaly detection and the corresponding engine power it gets from data analytics and intelligence. If that’s not an excuse to take our overalls off and sit down for a tea break, then nothing is.
- Telix's bid to Expand Access to Cancer RadiopharmaceuticalsProcurement & Supply Chain
- Samsung Biologics Secures US$1.24Bn Manufacturing DealProduction & Operations
- Three Women, Three Professionals, Three Vital PerspectivesProduction & Operations
- Why Stora Enso's new dry Forming Production Unit MattersSustainability & ESG