Smart data: resilience in manufacturing & the supply chain
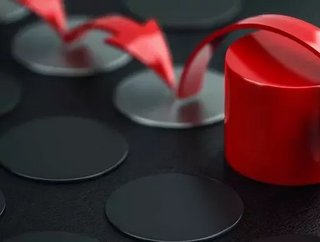
For many manufacturing and supply chain organisations, the Covid-19 pandemic highlighted multiple shortcomings in their data management strategies. Despite access to a wealth of data, they were often unable to use it to gain the overarching and accurate view of their business needed to plan for growth, make informed business decisions, and respond to disruptions.
This problem is rooted in processes and supporting technologies that have not been designed to work in unison. Systems generate data and produce reports independently, with the result that decisions are made separately, undermining efficiency.
Processes also become isolated from external data and workflows that could have a substantial impact on the business. This data includes the real-time data from sensors and other sources such as streaming services about weather, traffic or market conditions. The volume of this data has exploded, yet it still requires integration with batch data so that actionable insights are delivered fast and reliably in areas such as risk management.
The difficulty of achieving such data harmonisation means organisations distrust the data generated by their processes, putting them at a disadvantage. They lack the technology and expertise to extract the intelligence they need. Without an accurate overview of the business, it is difficult for manufacturers to plan for growth and practically impossible to respond to any disruptions. This is why many suffered extensive downtime as the pandemic took hold.
To gain resilience, agility, and faster more accurate decision-making capabilities with the aim of futureproofing operations, supply chain and manufacturing organisations must implement technologies to link silos in which data and processes sit.
The data lake does not take businesses all the way
The data lake approach has historically been put forward as the solution to this problem. On its own, however it has consistently failed to meet expectations. When demand for real-time insights increases, data lakes with their mixture of taxonomies, metadata and structures, have proved murky. It becomes difficult to integrate, normalise, and harmonise all the data so that organisations gain a consistent and comprehensive overview and are able to use it.
Further complexity is added because the torrents of real-time data must be harmonised alongside batch data. Through data-capturing devices (including IoT sensors), manufacturers and supply chain companies have access to real-time information about order status, the quality of product or raw materials, the current condition of their equipment, location of inventory and assets in transit and more. Such devices yield more data than any human being (and many existing systems) can manage, making it difficult for companies to extract insight and maximum value. The net result has been for the data lakes to become frozen – in effect, just another silo.
How smart data fabrics can effectively harmonising data
Companies cannot afford to neglect the integration of data, however. Organisations need new models of harmonisation that bring together disconnected processes, applications, and data. Above all, they must use the advances in data management technology delivered by ‘smart’ data fabrics, complemented by AI and machine learning (ML), along with new API-driven development approaches. By allowing existing applications and data to remain in place, smart data fabrics enable organisations to get the most from previous investments, extracting business value from data stored in lakes and external sources quickly and flexibly to power business initiatives. This includes everything from scenario planning to risk modelling.
The fabric intelligently connects and automates processes that cross existing system boundaries in a non-disruptive manner without having to ‘rip and replace’ existing or legacy systems. It interweaves disparate data, including real-time event data and data from supply chain partners, and allows for exposing, connecting, and orchestrating services and microservices. The result is a comprehensive and overarching perspective that enables frictionless interactions between functional areas and delivers greater flexibility and efficiency, and better insights led by AI.
Gaining these advantages from a data lake without a smart fabric would require multiple architectural layers, distributed data stores, an integration layer, transformation, normalisation, and harmonisation capabilities, a metadata layer, as well as a real-time, distributed caching layer. Then, there is also a need for an intelligence layer, with application logic and analytics capabilities, and a real-time layer. This is obviously complex and costly to build and maintain.
With businesses demanding more from the increasing levels of batch and real-time data they have available to them to gain increased efficiency and deliver value to customers, smart data fabrics are clearly the best route forward. This ability to leverage more data in the moment enables organisations to gain the vital capabilities they need to make better business decisions based on data, and in turn respond faster and better to crises while increasing revenue and reducing risk, especially during periods of disruption and volatility. It is also much cleaner architecturally, and simpler from an implementation, maintenance, and application development standpoint.
Making AI and ML part of the fabric
Advanced analytics technologies like AI and ML fuel a variety of use cases within the supply chain. One of the most significant is demand management. In this scenario, AI enables manufacturers to predict and model demand to manage situations proactively, rather than just reacting to them. While some organisations focus on aggregated demand forecasts based on historic data, those excelling in the field of AI have started to break down planning to a regional basis or to go down to individual customer requirements or products, using (near) real-time data. By conducting more detailed and accurate forecasting processes, manufacturers make significant improvements in performance and profitability.
Other use cases include sales and operations planning (S&OP) processes, which AI and analytics can transform by bringing together stakeholders and data from across sales, production, procurement, and other departments. This cross-departmental infusion of data can make a big difference to manufacturers and help them make more informed decisions, including a quicker response to fluctuations in demand, setting up promotions when production surpluses are projected.
Automation through AI embeds also smart decision-making that can include exception-handling. Not only is accuracy increased, but managers also gain time for more important tasks.
High performance, low latency and enhanced resilience
There is no longer a need for different development paradigms to manage the various application layers. The smart data fabric provides higher performance as latency is reduced due to the elimination of connections between the different layers of the architecture, allowing organisations to incorporate transaction and event data into analyses and processes in near-real-time.
With businesses fully aware they must fully exploit the high volumes of valuable data flowing into their organisations, the smart data fabric has come of age. It trumps the difficulties of unifying different types of data from many sources to provide telling insights that drive up the quality of critical decision-making and provide the firmest possible basis for future resilience and greater overall efficiency.
- SAP’s AI Joule set to Transform Supply Chain ManufacturingAI & Automation
- Top Ten: Diverse Companies in ManufacturingProduction & Operations
- How Smart Factories are Reshaping the Manufacturing SectorSmart Manufacturing
- Crowe UK: 2024 Manufacturing Outlook Report Explores GrowthProduction & Operations