Should Manufacturers be prioritising data analysis?
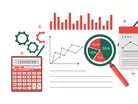
Poetically put, Jens Beck, Director for IIoT, Analytics, and Innovative Cloud Solutions at Syntax says “optimising the resources needed for a business to stay competitive is a challenge as old as business itself.” But coupling this with today’s soaring prices for raw materials, global competition, talent wars, and shifting customer expectation, “it is of utmost urgency for manufacturers to start using their data to optimise processes and business outcomes in order to stay competitive,” adds Beck.
“Privately, nearly everybody has already arrived in a super digital world, from smart washing machines to smart fridges, speech control and so forth, so why do we have to use paper and spare parts on stock at work? Why do our machines not talk to each other; why do they not tell me when they need maintenance; why do they not tell my service provider in advance when to come and what spare parts to bring? This is simply because digitisation on the shop floor has only just begun. The source to make this all happen is data and especially the digital twin.”
But how close is this dream within reach? Francois Zimmermann EMEA Field CTO, Tableau Software explains that despite “87% of CXOs saying that being an intelligent enterprise is their top priority, the reality is that only 30% of frontline employees’ actions are driven by data analysis. This means that most business decisions are made based on bias or on what has worked in the past rather than live actionable insights.”
With leaders in manufacturing under pressure to identify risks in complex supply chains that extend beyond their own boundaries, while achieving sustainability goals, succeed, and retain their customers, “leveraging the right data, can create a free flow of the facts that they need to make critical and profitable decisions, while at the same time, increasing visibility and resilience across their supply chains,” adds Zimmermann.
By adopting a data first strategy, manufacturers can look at every key business activity, ensuring that all stakeholders have access to a 360 degree view of their customers and operations in order to make effective decisions fast. “In manufacturing this becomes an extension of design thinking in product design, so that fast feedback loops are created to continuously improve products and processes,” he continues.
“By gathering data at every opportunity and examining workflows, points of inefficiency can be quickly identified and the real issues exposed. A data-first strategy promotes looking under the hood of the business to assess in granular detail where time and money is being wasted and where weak points might present a security risk. Then, insights and predictions can be applied to tweak operations and workflows where changes will have a positive impact, without spending money on solutions when they are not necessarily needed.”
Successfully adopting a data first strategy
Both agreeing on what constitutes a data strategy, Beck and Zimmermann both agree that a successful data-first strategy encompasses both culture and technology.
“In my opinion, the foremost important one is the right mindset. Trust your data, work on your data quality, establish data governance, so if you have not done so yet – start collecting data. A successful data-first strategy is not brought to life overnight, it is a journey. Start with small overseeable pilots to build and gain trust in your data across your organisation. As these first successes continue, convince people of the benefits of using their adequate data,” says Beck.
Expanding on this, Zimmermann adds: “manufacturers that want to build a data culture need to address data governance and data literacy in order to enable stakeholders to access trusted data assets from across the whole business and answer new questions without over-reliance on core IT.
“To deliver an agile data foundation, manufacturers should look to create a single source of truth that pulls together data from across the entire business. They can leverage low-code or no-code, cloud-based, SaaS data management and analytics platforms to enable more parts of the business to self-serve data and insights.
Manufacturers should then look for ways to bring those insights into key business apps so that front-line workers can use them as a seamless part of their workflow.”
Enter, predictive analytics…
“Per definition, predictive analytics tells you what is going to happen next based on trained models and historical data – so by referencing a past experience,” says Beck, which is why he defines it as “the most valuable use case.”
Predictive analytics assesses workflows and finds inefficiencies to predict future events i.e. How might a supply chain hold up under X or Y conditions? What points of weakness might create issues under specific sets of circumstances?
“Using historical and real-time data, predictive analytics can bring to light possible future outcomes and identify potential issues that manufacturers can then preemptively mitigate. This enables manufacturers to make data-driven decisions about their business with full end-to-end visibility,” adds Zimmermann.
How can predictive analytics help manufacturers to improve their operations?
By harnessing predictive analytics, manufacturers can improve their operations in a number of ways; improving overall equipment efficiency, improving shop floor efficiency, optimising production planning, improving safety, improved productivity, improving quality, and mitigating risks.
“Two key areas where predictive analytics can help manufacturers improve their operations are reducing risks in the supply chain and driving sustainability,” says Zimmermann. “Supply chains naturally generate huge amounts of data, which is key to building the data sets needed for predictive analytics. With so many elements and variables, risks in the supply chain are inevitable. With predictive analytics it is possible to identify these before they have an impact, and even test what the results of a decision would be before it is made. This can improve safety measures for staff, and empower manufacturers to make rapid changes to business operations in the face of disruption without fear of hidden risks and negative outcomes.
“Increasingly, sustainability in manufacturing is critical. This is driven not only by customer demand for sustainable products and packaging, but also the rising cost of energy and raw materials. But the global supply chain remains extremely inefficient. While manufacturing accounts for 50% of the gross world product (GWP), 20% of every dollar the sector spends is wasted. Put simply,10% of everything made worldwide is lost to waste and inefficiencies. Predictive analytics can identify these inefficiencies, helping manufacturers to improve yield, uptime and time to market, boosting productivity significantly while helping manufacturers to achieve their carbon footprint goals.”
What will the future hold for predictive analytics?
Over the years, predictive analytics technology has rapidly improved, reaching a mature and simplified state where manufacturers “do not necessarily need data scientists to walk our first steps in the direction of predictive analytics,” says Beck.
“Hyperscalers have made the technology available for affordable prices and a lot of solutions and products are readily available on the market. All of this development has a knock-on effect on industrial sensors, IIoT gateways, networks and so forth, where the prices also came down while services, availability and capabilities became available. Take the development of 3G, 4G and 5G, in combination with cloud computing, which only enables topics like smart farming or smart cities. On the downside, the number of providers and solutions is growing so fast that it is very difficult for a customer to get an overview and find the right solution without being an expert.”
When it comes to the future for this technology, Beck adds: “I currently see two evolvements for the future of analytics technology. First there are more and more micro-services, solutions and products to allow normal users to tap into the field of predictive analytics without special skills or pre-knowledge. Even though the risk and weakness of these offerings is that they are not considering the totality of the overall picture, they help educate people, build trust and are good starters for a data-first strategy. It's important for organisations to find the right balance when engaging with somebody to develop the solution path for their specific scenario.
“Secondly, I see strong evolution bringing predictions and big data analytics back to the edge at reasonable cost. This will be very interesting to see since it will open up even more business cases where high network traffic costs often hinder profitable business cases. The next major evolution will be affordable quantum technology which allows even larger prediction models and will truly be the enabler for complex prescriptive analytics.”
Echoing Beck's comments, Zimmermann also adds, “the most significant change affecting predictive analytics and its future is its democratisation. Today data science is often deeply technical, expensive, hard to scale and siloed from the rest of the business. Low-code and no-code platforms are bringing these capabilities closer to line of business users that understand their workflow and have domain expertise but may not have data science training. This is key to improving time to value and ensuring that predictive analytics can deliver value at the point of maximum impact.”
Zimmermann sees the quick wins being in the augmented experiences that bring predictive insights directly to data consumers. “Business users will need a variety of experiences that empower them to work alongside an AI to derive value from their data assets. The best tools can quickly and efficiently pull together large data sets from multiple sources into an analytics workbench that lets users understand ‘what has happened and why’, ‘what will happen’, and ‘what is likely to happen if a specific action is taken’. This enables business users to sift through massive data sets and explore millions of combinations in seconds to automatically surface insights. If users can build custom predictions and perform what-if analysis, they can then bring these models and recommendations into their business workflow to operationalise the findings for the whole organisation. The future of predictive analytics is therefore that more data consumers can effectively see and understand their data.”
- Digitalisation as a Strategy to Attract and Retain WorkersDigital Factory
- UPDATED VENUE & DATE – Manufacturing LIVE Chicago 2025Sustainability & ESG
- ABB Cuts Industrial E-Waste in Quest for CircularitySustainability & ESG
- Why Are Manufacturers Doubling Down On Digital Twins?Digital Factory