Understanding artificial intelligence (AI) in manufacturing
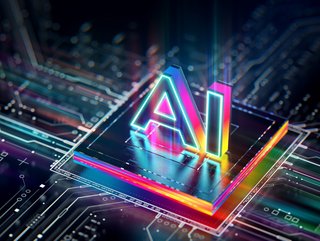
Research conducted by Accenture on the impact of artificial intelligence (AI) in manufacturing has revealed that the technology could add an additional US$3.8 trillion gross value added (GVA) by 2035, with a rise in profitability of 38%.
As such, manufacturers are keen to implement new solutions to help drive their business forward and according to McKinsey the adoption of AI in businesses more than doubled between 2017 and 2022.
What is fueling the adoption of AI?
Much of this growth is being fuelled by the ongoing roll-out of internet of things (IoT) and connected devices – a natural precursor to the introduction of AI in industry. AI needs data on which to perform operations, and IoT provides a ready source. By analysing data streams from sensors across an IoT infrastructure, AI can be used to build a picture of manufacturing processes, and then suggest automation or improvements based on various optimisation goals.
But machines do not operate in a silo, and so in order to realise this potential, AI also needs to be both dependable and explainable, as well as intuitive. For example, embedding AI in forecasting capabilities can significantly improve demand accuracy. However, on the flip side of this, humans also have to be able to interact with AI and query it, in order to understand the reasons for decisions that have been suggested and ensure that the progress made is used for the benefit of the organisation itself.
What is explainable AI and how does it improve accountability for the technology?
AI’s rapid expansion is backed by huge investments, based on its promise of delivering so many potential commercial benefits to businesses, not least within the manufacturing sector. By examining how processes are undertaken, AI can learn how to make improvements. Part of this is likely to involve reducing the amount of user involvement, but at the same time, AI cannot, nor should not, run in complete isolation from humans.
AI, therefore, always has to be explainable. After all, if a human is required to have the final sign-off on a critical business process, they need to understand what they are signing. That means the results need to be presented in a way that is easily intelligible. Still, more importantly, every process needs to be auditable – and that will also necessitate human involvement. Signing off on a new process may have impacts on safety. If a machine in the manufacturing process runs hot and creates a fire, it will be a human safety officer, and not the AI, who will ultimately need to take responsibility.
While AI and automation are lightly regulated at the moment, there are increasing calls for this to change. It is almost certain that businesses will need to provide some kind of traceable log to show why decisions were made the way that they were. Logging creates data that can be used to learn lessons for the future. This is a new area that is not currently covered by legislation or frameworks but is nevertheless coming, and it is critically important that businesses prepare themselves for it. While some AI models can show sources or calculations for their output, others more resemble a black box.
Explainability feeds into this, in that organisations will have to provide output from the decisions in a way that a human can interpret.
How can organisations ensure that data is easily understood?
Sometimes even straightforward decisions are going to be based on incredibly large volumes of complex data. That, in a nutshell, is the value of data streaming. But the sheer volume of data involved in real-time streaming means that no human would be able to make sense of it in its rawest form.
Explainable AI is, therefore, becoming an increasingly prominent concept. Essentially, it is a set of processes and methods, a protocol of sorts, that allows human users to understand and trust the results and output created by machine learning algorithms. Explainable AI delivers an array of benefits from meeting regulatory standards, helping developers ensure the system is dependable, working well and enabling people impacted by a specific decision to subsequently challenge it.
Accordingly, we are increasingly seeing more demand for artificial intelligence technology that works to this protocol, allowing the output to be forensically examined by humans to understand the rationale for its decisions.
In this sense, it is extremely important that manufacturing organisations understand who trains their AI systems, what data was used and, just as importantly, what went into their algorithms’ recommendations. A high-quality explainable AI system will deliver this for them.
What does the future look like for manufacturers?
The use cases for AI within the manufacturing sector are already vast and will continue to multiply in the future, particularly as they become more case driven. As more and more data is created in the manufacturing process within smart factory environments, new applications will inevitably evolve. But reliance should not be placed on AI that has no ability to explain or justify its actions, and the easier it can express itself, the easier it will be for humans to understand its decision-making, regulate it and have oversight of processes to retain responsibility for outcomes.
- Exotec: Warehouse Automation & The British Alcohol IndustrySmart Manufacturing
- SAP’s AI Joule set to Transform Supply Chain ManufacturingAI & Automation
- Top Ten: Diverse Companies in ManufacturingProduction & Operations
- EY Report Shows AI Being Adopted in Advanced ManufacturingAI & Automation